Denoising
[1]:
import spectrochempy as scp
|
SpectroChemPy's API - v.0.7.1 © Copyright 2014-2025 - A.Travert & C.Fernandez @ LCS |
Denoising 2D spectra
Denoising 2D spectra can be done using the above filtering techniques which can be applied sequentially to each rows of a 2D dataset.
e.g., let’s take a series of Raman spectra for demonstration: These spectra present both a significant noise and cosmic rays spikes.
[2]:
# Load the data ( )
dataset = scp.read("ramandata/labspec/serie190214-1.txt")
# select the useful region (in particular spectra are 0 after 6500 s)
nd = dataset[0.0:6500.0, 70.0:]
# baseline correction the data (for a easier comparison)
nd1 = nd.snip()
# plot
prefs = nd1.preferences
prefs.figure.figsize = (9, 5)
_ = nd1.plot()
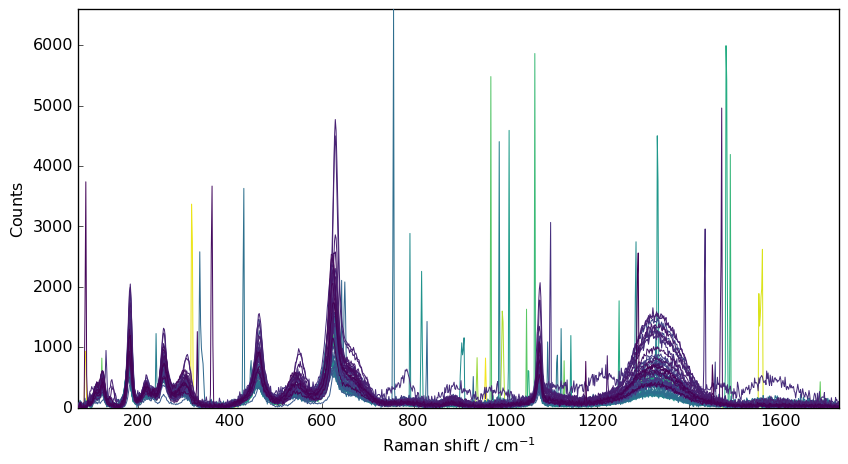
We can apply a Savgol filter to denoise the spectra
[3]:
nd2 = nd1.savgol(size=7, order=2)
_ = nd2.plot()
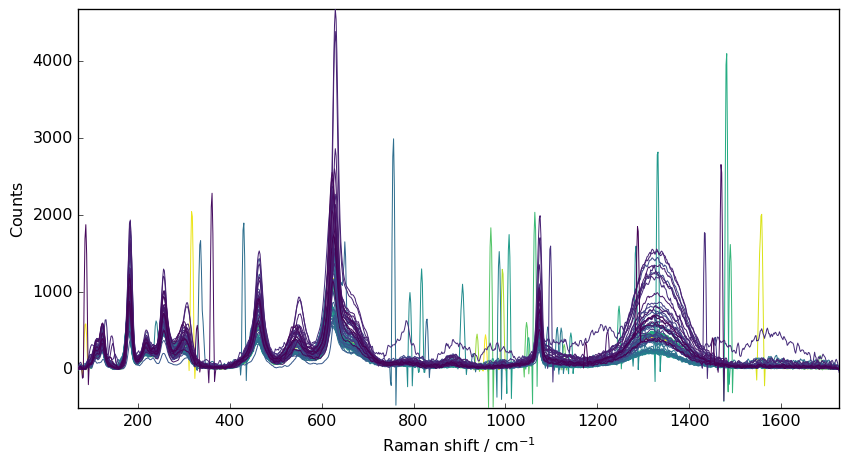
The problem is that, not only the spikes are not removed, but they are also broadened.
A better way to simply denoise this spectra is to use the denoise
dataset method.
The ratio parameter fix the amount of variance we want to preserve in % (default 99.8%)
[4]:
nd3 = nd1.denoise(ratio=90)
_ = nd3.plot()
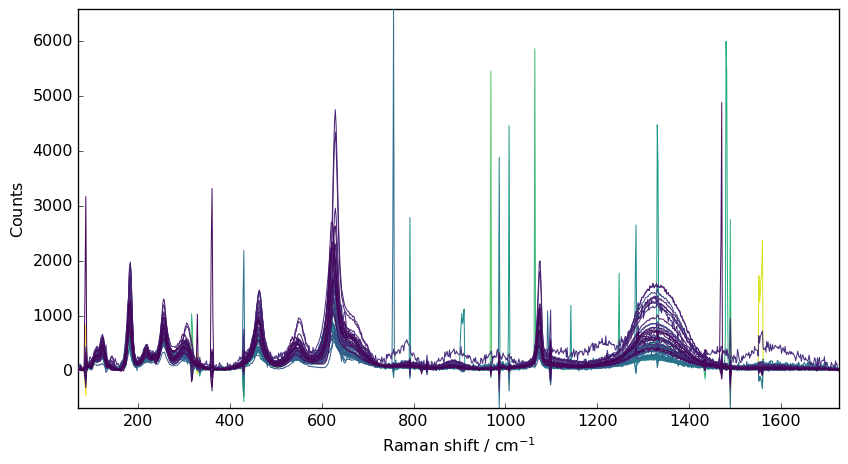
This clearly help to increase the signal-to-noise ratio. However, it apparently has in the present case a poor effect on eliminating cosmic ray peaks.
Removing cosmic rays spike from Raman spectra
Median filter
A first way to perform this is to apply a median-filter to the data
[5]:
filter = scp.Filter(method="median", size=5)
nd4 = filter(nd1)
_ = nd4.plot()
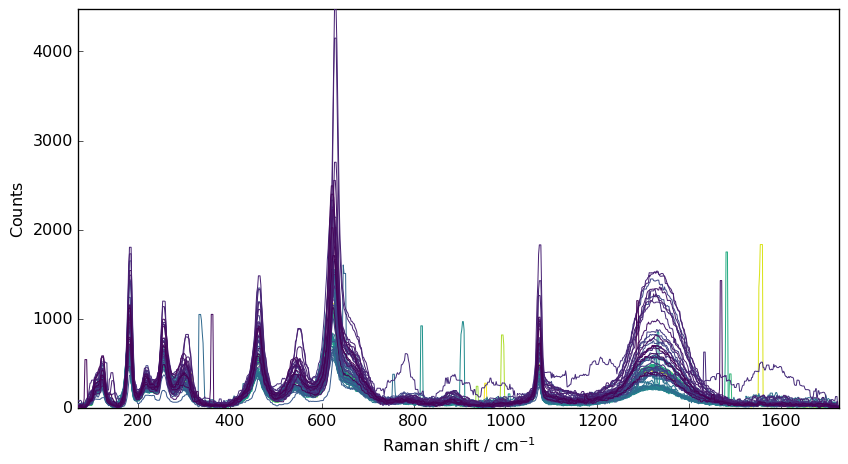
However, the spike are not fully removed, and are broadened.
despike
method
To obtain better results, one can use the despike methods. The default method (‘katsumo’) is based on :cite:t:katsumoto:2003
. The second one (‘whitaker’) is based on :cite:t:Whitaker:2018
For both methods, only two parameters needs to be tuned: delta
, a threshold for the detection of spikes, and size
the size of the window to consider around the spike to estimate the original intensity.
[6]:
X = nd1[0]
nd5 = scp.despike(X, size=11, delta=5)
_ = X.plot()
_ = nd5.plot(clear=False, ls="-", c="r")
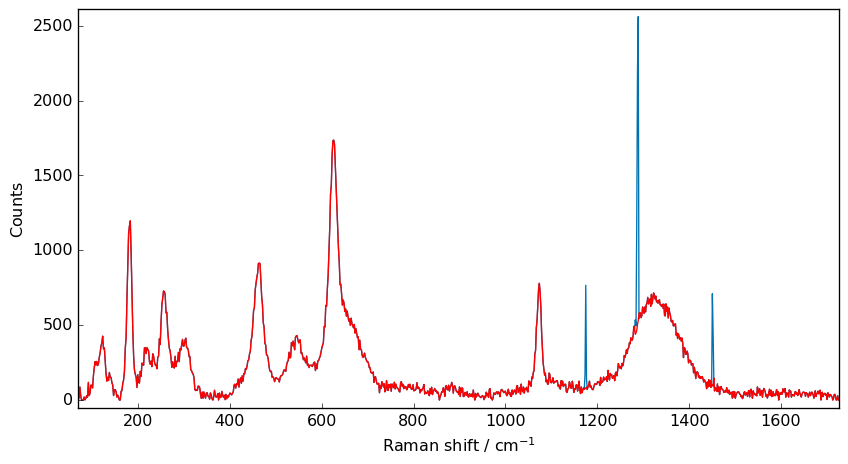
Getting the desired results require the tuning of size and delta parameters. And sometimes may need to repeat the procedure on a previously filtered spectra.
For example, it size or delta are badly chosen, valid peaks could be removed. So careful inspection of the results is crucial.
[7]:
nd5b = scp.despike(X, size=21, delta=2)
_ = X.plot()
_ = nd5b.plot(clear=False, ls="-", c="r")
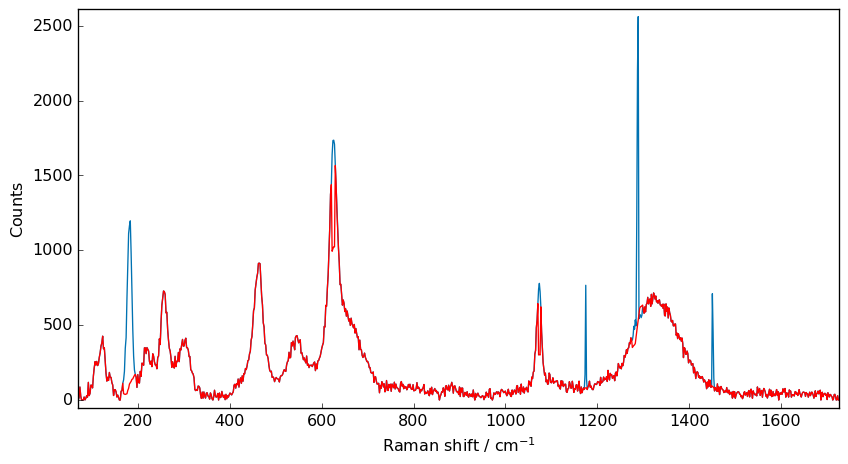
Last we can apply it to the full 2D dataset
[8]:
nd6 = scp.despike(nd1, size=11, delta=5)
_ = nd6.plot()
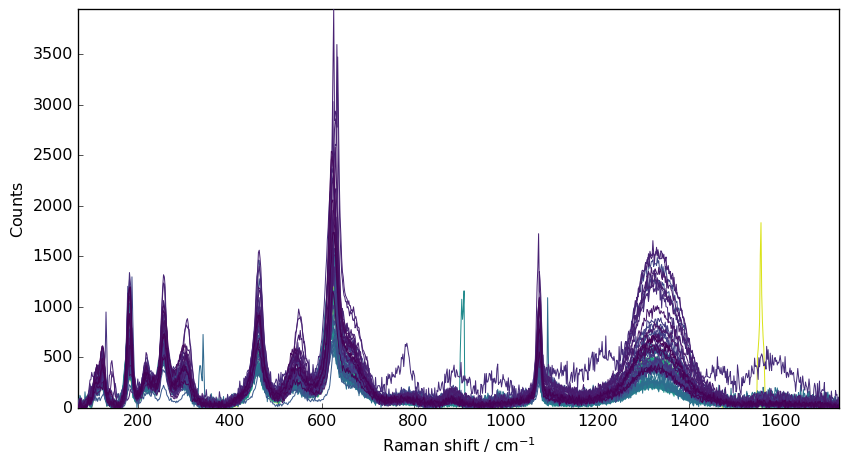
It is however rarely perfect as the setting of size and delta may be depending on the row.
A possibility to improve it is to apply a denoise filter afterward.
[9]:
nd7 = nd6.denoise(ratio=92)
_ = nd7.plot()
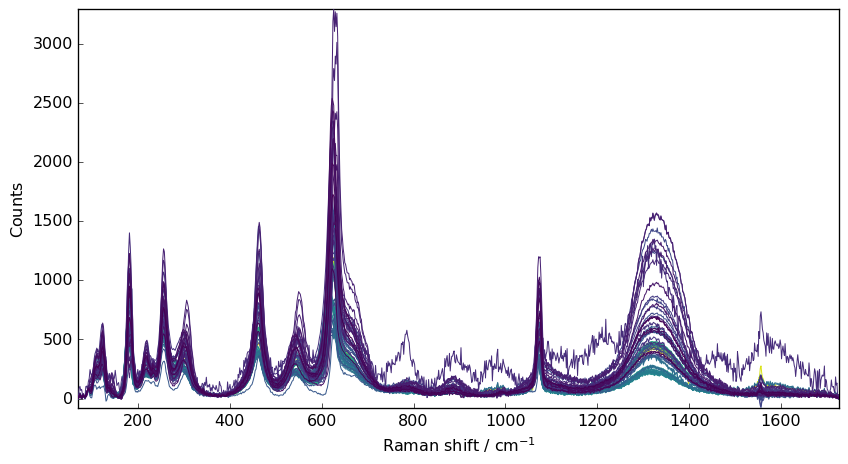
The ‘whitaker’ method is also available:
[10]:
nd8 = scp.despike(nd1, size=11, delta=5, method="whitaker")
_ = nd8.plot()
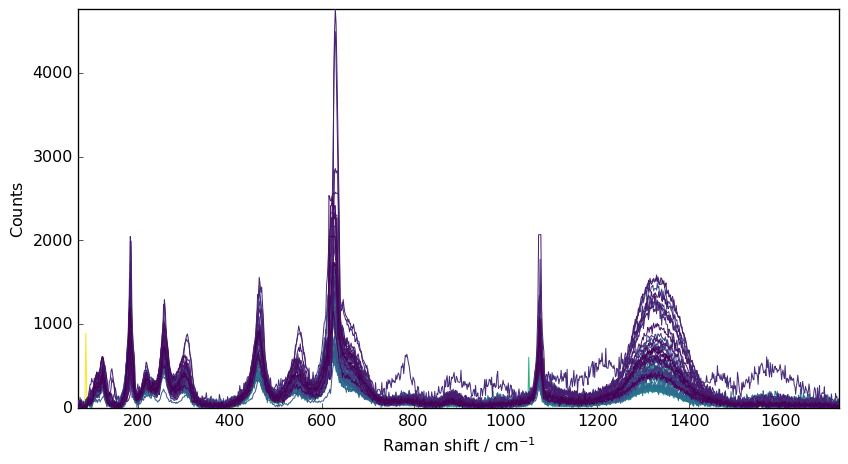